You signed in with another tab or window. Reload to refresh your session.You signed out in another tab or window. Reload to refresh your session.You switched accounts on another tab or window. Reload to refresh your session.Dismiss alert
기본적으로 autoregressive 모델은 train 할 때나 test 할 때나 앞에서부터 순서대로 inference 를 수행하게 된다.
하지만 그럴 필요 있을까?
아래 그림을 보면 (3, 1, 2, 4) 순으로 prediction을 수행한다. 회색 동그라미는 모두 shared layer 이다. 즉, shared layer 들에 autoregressive 하게 기존과는 다른 순서로 inference 를 하는 것이다. 당연하게도, 다음 inference 를 수행할 때는, 이전 output 들을 사용한다.
inference 결과물을 보면 아, 이런식으로 하는 거구나 싶다.
ARDM
모델은 transformer encoder, 786 dim, 12 layer, 12 head
inference 순서는 random order 순이다.
inference 로직은 다음과 같이 단순하다 m mask 는 t time-step 이전의 output 들만을 보게 해 주는 mask 이고, n mask 는 output 에서 원하는 특정 target output 을 제외한 녀석들을 mask 시켜주는 녀석이다.
training 시킬 때는 약간 다르다.
특정 time-step t 를 뽑아내고 t 개의 mask 를 만들어 내서, 한 번에 예측하도록 한다. BERT 의 MLM 과 굉장히 유사하다.
Parallellized ARDM
inference 할 때 하나씩 하는 건 느리니까 한 번에 묶어서 prediction 을 하는 실험도 진행해 보았다.
아래 왼쪽 그림을 보면 시간이 지날수록 generation loss 가 줄어 든다는 것을 알 수 있다.
그니까, 왼쪽에서는 20번 inference 가 수행된 건데,
오른쪽에서는 (1, 2, 4, 8, 16) 으로 5번 inference 한 것이다.
(1, 2, 4, 8, 16) 을 얘네가 그냥 정한 건 아니고, 학습 데이터들의 Loss data 를 쭉 뽑아두고 Dynamic Programming 을 적용해서 뽑는다.
해당 set 에서 Loss step 을 어느 정도로 묶어줘야 Loss 가 가장 작아질 수 있을 지, 미리 뽑아내는 느낌.
당연히 성능은 떨어지지만, 그래도 inference speed 가 많이 빨라지는 것을 생각하면, 꽤 좋다.
Depth Upscaling ARDM
pixel color (255개 pixel classification) 를 예측하는 문제를 생각해 보자.
이것을 여러 step으로 차근차근 풀어낼 수 있다.
1-step : 128 보다 낮은지, 낮지 않은지 풀어내기
2-step : (1-step) 에서 128 보다 낮은 녀석들은 64를 기준으로 pixel 값이 높은지, 낮은지 풀기. 마찬가지로 (1-step)에서 128보다 높았던 애들은 196을 기준으로 계산
이렇게 풀면 8-step 이면 최종 pixel 값을 구할 수 있다.
visualize 하면 이런 느낌.
Limitiation
논문에서 직접 말하고 있는 limitation.
아직 다양한 task 에서 single-order autoregressive 모델보다 성능이 딸린다.
ARDM은 discrete variable 을 모델링한다. continuous distribution 으로의 확장이 필요하다.
Cross-Entropy 밖에 안 써봤다. 다양한 architecture와 loss 들이 실험되어야 한다.
Results
The text was updated successfully, but these errors were encountered:
paper
yannic youtube
기본적으로 autoregressive 모델은 train 할 때나 test 할 때나 앞에서부터 순서대로 inference 를 수행하게 된다.
하지만 그럴 필요 있을까?
아래 그림을 보면 (3, 1, 2, 4) 순으로 prediction을 수행한다. 회색 동그라미는 모두 shared layer 이다. 즉, shared layer 들에 autoregressive 하게 기존과는 다른 순서로 inference 를 하는 것이다. 당연하게도, 다음 inference 를 수행할 때는, 이전 output 들을 사용한다.

inference 결과물을 보면 아, 이런식으로 하는 거구나 싶다.

ARDM
모델은 transformer encoder, 786 dim, 12 layer, 12 head
inference 순서는 random order 순이다.
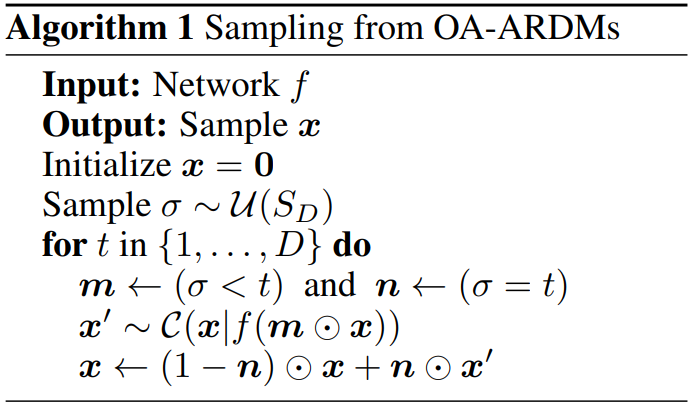
inference 로직은 다음과 같이 단순하다
m
mask 는 t time-step 이전의 output 들만을 보게 해 주는 mask 이고,n
mask 는 output 에서 원하는 특정 target output 을 제외한 녀석들을 mask 시켜주는 녀석이다.training 시킬 때는 약간 다르다.
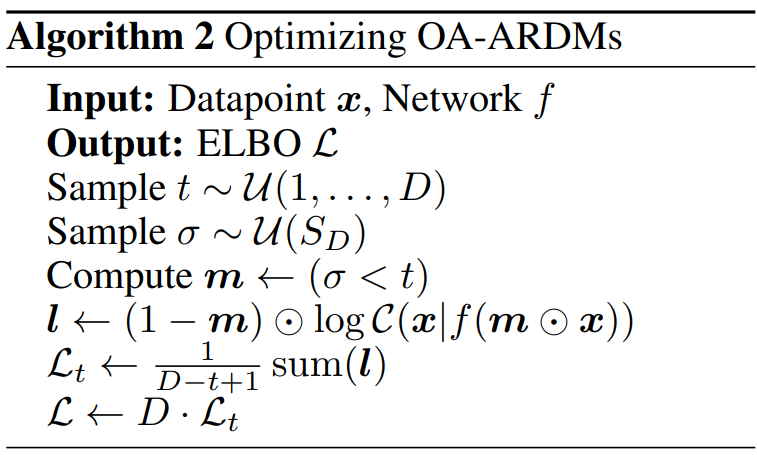
특정 time-step t 를 뽑아내고 t 개의 mask 를 만들어 내서, 한 번에 예측하도록 한다. BERT 의 MLM 과 굉장히 유사하다.
Parallellized ARDM
inference 할 때 하나씩 하는 건 느리니까 한 번에 묶어서 prediction 을 하는 실험도 진행해 보았다.

아래 왼쪽 그림을 보면 시간이 지날수록 generation loss 가 줄어 든다는 것을 알 수 있다.
그니까, 왼쪽에서는 20번 inference 가 수행된 건데,
오른쪽에서는 (1, 2, 4, 8, 16) 으로 5번 inference 한 것이다.
(1, 2, 4, 8, 16) 을 얘네가 그냥 정한 건 아니고, 학습 데이터들의 Loss data 를 쭉 뽑아두고 Dynamic Programming 을 적용해서 뽑는다.
해당 set 에서 Loss step 을 어느 정도로 묶어줘야 Loss 가 가장 작아질 수 있을 지, 미리 뽑아내는 느낌.
당연히 성능은 떨어지지만, 그래도 inference speed 가 많이 빨라지는 것을 생각하면, 꽤 좋다.

Depth Upscaling ARDM
pixel color (255개 pixel classification) 를 예측하는 문제를 생각해 보자.
이것을 여러 step으로 차근차근 풀어낼 수 있다.
1-step : 128 보다 낮은지, 낮지 않은지 풀어내기
2-step : (1-step) 에서 128 보다 낮은 녀석들은 64를 기준으로 pixel 값이 높은지, 낮은지 풀기. 마찬가지로 (1-step)에서 128보다 높았던 애들은 196을 기준으로 계산
이렇게 풀면 8-step 이면 최종 pixel 값을 구할 수 있다.
visualize 하면 이런 느낌.
Limitiation
논문에서 직접 말하고 있는 limitation.
Results
The text was updated successfully, but these errors were encountered: